Scala/Spark Expert-Scala and Spark code assistant
AI-Powered Scala and Spark Engineer

Expert assistant in Scala and Spark for data engineering tasks.
How do I optimize a Spark job?
Explain a Scala function in Spark context.
Best practices for data partitioning in Spark?
Troubleshooting a Scala code issue in Spark.
Get Embed Code
Overview of Scala/Spark Expert
Scala/Spark Expert isScala Spark Expert Overview a specialized AI assistant tailored for advanced data engineering tasks using Apache Spark and the Scala programming language. It is designed to assist data engineers, software developers, and big data practitioners by offering in-depth support for distributed data processing, performance optimization, and robust Scala code development. Unlike general-purpose AI tools, Scala/Spark Expert focuses on delivering practical, code-driven, and scenario-specific insights rooted in real-world Spark and Scala development practices. For instance, if a user is facing a performance bottleneck in a Spark job due to an inefficient join operation on large datasets, Scala/Spark Expert would not only suggest optimized join strategies (e.g., broadcast joins, bucketing) but also provide example code snippets and DAG inspection tips to improve performance. Similarly, for a user designing a typed dataset transformation pipeline in Scala, this expert offers precise, type-safe implementation techniques using case classes, implicits, and functional programming constructs native to Scala.
Core CapabilitiesScala Spark Expert Overview and Applications
Spark Performance Optimization
Example
A data engineer runs a Spark job that performs several wide transformations (e.g., joins and aggregations) on terabyte-scale data. The job is slow and prone to executor memory issues.
Scenario
Scala/Spark Expert would analyze the likely causes (e.g., skewed keys, improper partitioning, lack of caching), and suggest techniques like salting, repartitioning, or using broadcast joins. It would also provide code examples and advice on inspecting Spark UI stages and metrics to validate improvements.
Typed Data Pipelines Using Scala
Example
A developer wants to process structured data using Spark's Dataset API while retaining compile-time type safety and leveraging functional programming idioms.
Scenario
Scala/Spark Expert assists in designing case class models, leveraging `map`, `flatMap`, `filter`, and other higher-order functions efficiently. It shows how to use custom encoders, manage nullability safely, and apply transformations in a type-safe and testable way.
Advanced Spark Job Design and Refactoring
Example
A team is refactoring a monolithic ETL job written in PySpark into a modular, maintainable Scala Spark application.
Scenario
Scala/Spark Expert guides in modularizing code using traits and abstract classes, defining reusable UDFs/UDAFs in Scala, handling configuration with Typesafe Config, and structuring the project using sbt. It provides code templates and best practices for clean, testable, and efficient Spark codebases.
Target Audience and Beneficiaries
Data Engineers Working with Big Data Pipelines
These users typically handle ETL/ELT workflows on large datasets using Apache Spark. Scala/Spark Expert supports them by offering optimized approaches to data transformations, job orchestration strategies, and memory/performance tuning. They benefit from actionable, performance-aware suggestions and type-safe Scala patterns that reduce runtime errors and improve code reliability.
Scala Developers Building Distributed Applications
Scala developers working in domains like real-time analytics, ML preprocessing, or batch pipelines find value in Scala/Spark Expert's deep integration with functional and type-safe coding paradigms. It helps them write expressive, concise code and debug complex Spark applications more effectively, especially when transitioning from local development to cluster execution.
How to Use Scala/Spark Expert
1. Access the Tool
Visit aichScala Spark Expert Guideatonline.org for a free trial without login; ChatGPT Plus is not required. Simply open the site and start using the tool immediately.
2. Define Your Task
Clearly identify whether your task is Spark-specific (e.g., writing a DataFrame transformation pipeline), Scala-focused (e.g., working with functional collections), or a combination. The more precise your input, the more relevant the output.
3. Interact in Technical Language
Communicate using code-level queries, design patterns, or problem statements in Spark/Scala. The expert understands context like RDDs, DAGs, lazy evaluation, Catalyst, and type-safe collections.
4. Iterate with Feedback
Use follow-up queries to refine or expand answers—Scala/Spark Expert handles stateful dialogue, allowing you to debug, optimize, or rework pipelines in iterative steps.
5. Apply Best Practices
Request or verify performance tuning tips (e.g., avoiding wide transformations, using broadcast joins)Scala Spark Expert Guide and architectural suggestions to build robust and scalable Spark/Scala applications.
Try other advanced and practical GPTs
MS Access Copilot
AI-powered assistant for Microsoft Access users

Lexi Uncensored
Unfiltered AI that tells it straight.

Image Wizard 🧙♂️✨
Turn your ideas into art—instantly, with AI magic.
Psychoanalytic Therapy Gestalt Therapist (non-med)
AI-powered self-exploration using therapy insights

AI Image Extender Free
Effortlessly resize and extend images with AI.
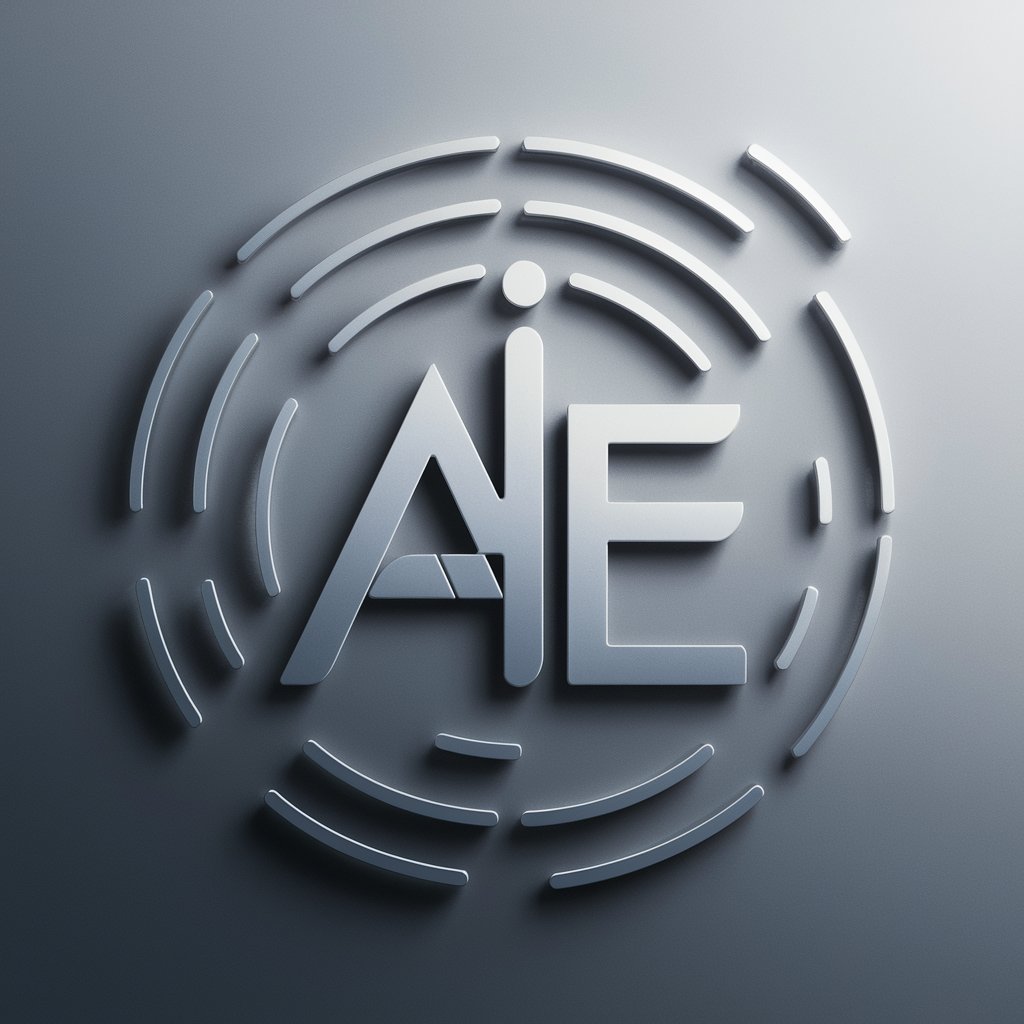
Research Poster Maker
AI-powered tool for compelling research posters
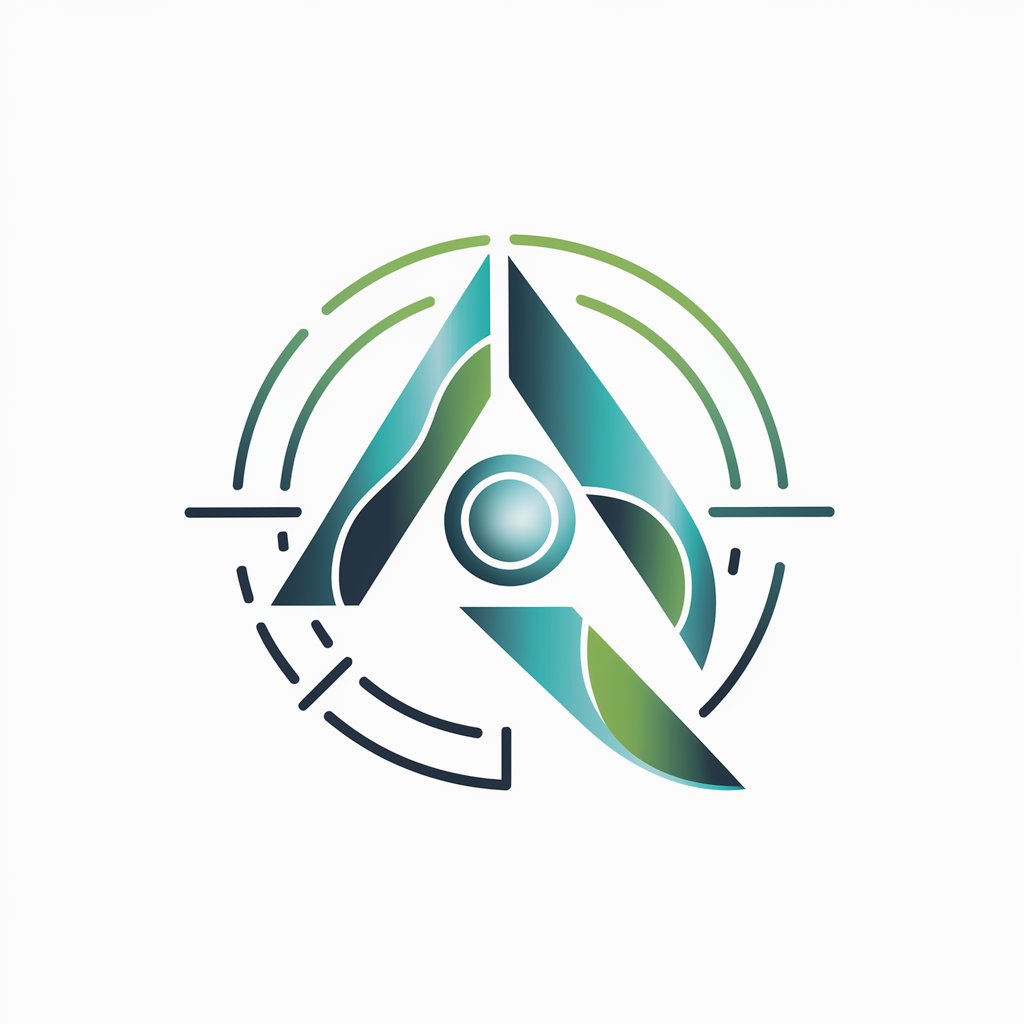
幻想的なアニメ風イラスト生成AI
Create stunning anime fantasy worlds with AI

Music Prompt Generator ✨
AI-crafted lyrics with matching cover art
GPT who doesn't talk much
AI with attitude—no sugarcoating, no mercy.

Website Builder [Multipage & High Quality]
AI-powered builder for beautiful multipage sites
📷 Camera Gear Consultant: Video, Camera, Drone
AI-powered gear expert for cameras, video, and drones
Interactive Romance Novel Creator PRO
AI-powered stories where you write your heart’s path.
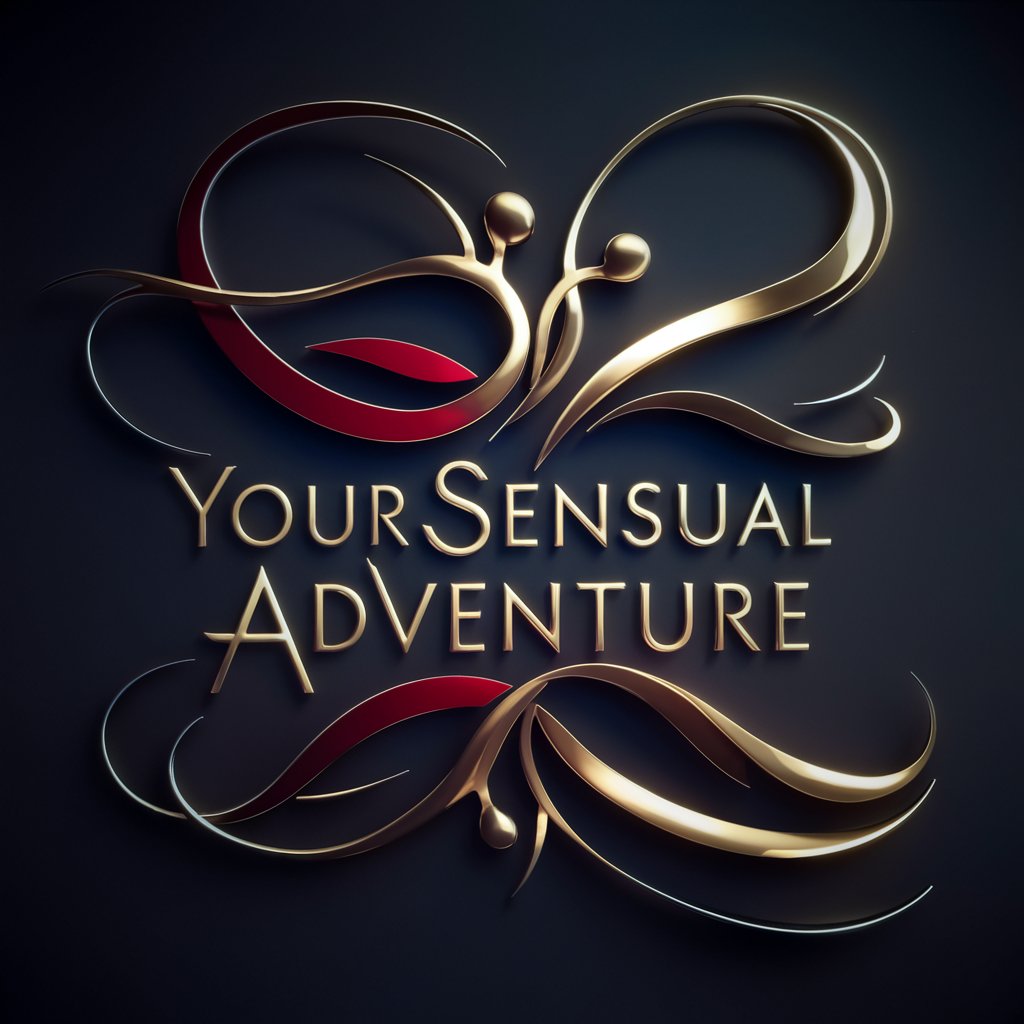
- Code Debugging
- Concept Explanation
- Query Optimization
- Pipeline Design
- System Integration
Frequently Asked Questions About Scala/Spark Expert
What kind of tasks can Scala/Spark Expert help with?
It supports a wide range of data engineering needs—writing Spark SQL queries, building ETL pipelines, optimizing transformations, debugging jobs, understanding error messages, implementing type-safe functional Scala code, and integrating with technologies like Delta Lake, Hive, and Kafka.
Can Scala/Spark Expert generate complete production-ready code?
Yes, it can generate modular, idiomatic, and production-grade code with comments, best practices, and performance considerations tailored to your use case—be it batch jobs with Spark or real-time Scala microservices.
How does it handle Spark performance tuning?
It offers guidance on tuning Spark configurations (e.g., `spark.sql.shuffle.partitions`, executor memory settings), joins (broadcast vs. sort-merge), data partitioning strategies, and code refactoring to avoid shuffles and improve DAG execution.
Is it suitable for learning or only for professionals?
Both. Beginners get detailed, step-by-step help with explanations of complex concepts like closures, lazy evaluation, Catalyst optimization, or implicits. Advanced users get help with architectural decisions, optimization strategies, and system-level integration.
Does it understand context from earlier parts of the conversation?
Yes. It keeps track of ongoing technical discussions, prior code snippets, and architectural choices. This makes it effective for complex problem-solving over long sessions.